XGBoost¶
XGBoost is an optimized distributed gradient boosting library designed to be highly efficient, flexible and portable. It implements machine learning algorithms under the Gradient Boosting framework. XGBoost provides a parallel tree boosting (also known as GBDT, GBM) that solve many data science problems in a fast and accurate way. The same code runs on major distributed environment (Kubernetes, Hadoop, SGE, MPI, Dask) and can solve problems beyond billions of examples.
Logo |
![]() |
---|---|
Website |
|
Repository |
|
Byline |
Scalable, Portable and Distributed Gradient Boosting (GBDT, GBRT or GBM) Library, for Python, R, Java, Scala, C++ and more. Runs on single machine, Hadoop, Spark, Flink and DataFlow. |
License |
Apache 2.0 |
Project age |
8 years 10 months |
Backers |
AWS (Sponsored by), Distributed (Deep) Machine Learning Community @ University of Washington (Creator and maintainer), Intel (Sponsored by), NVIDIA (Sponsored by) |
Lastest News (2022-10-31) |
Release 1.7.0 stable We are excited to announce the feature packed XGBoost 1.7 release. Some major improvements include: initial support … more |
Size score (1 to 10, higher is better) |
6.25 |
Trend score (1 to 10, higher is better) |
5.25 |
Education Resources¶
URL |
Resource Type |
Description |
---|---|---|
Documentation |
Official project documentation. |
Git Commit Statistics¶
Statistics computed using Git data through November 30, 2022.
Statistic |
Lifetime |
Last 12 Months |
---|---|---|
Commits |
70,545 |
4,678 |
Lines committed |
13,831,096 |
830,238 |
Unique committers |
589 |
61 |
Core committers |
10 |
9 |
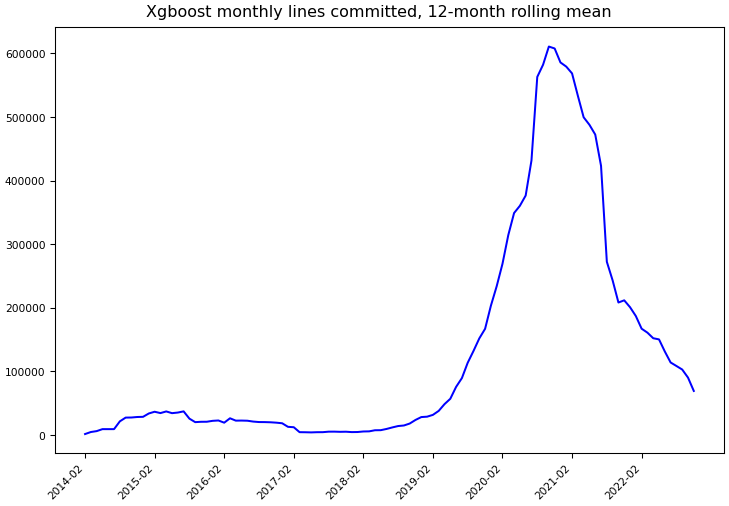
Similar Projects¶
Project |
Size Score |
Trend Score |
Byline |
---|---|---|---|
9.25 |
5.75 |
A fast, scalable, high performance Gradient Boosting on Decision Trees library, used for ranking, classification, regression and other machine learning tasks for Python, R, Java, C++. Supports computation on CPU and GPU. |
|
3.25 |
5.5 |
A collection of state-of-the-art algorithms for the training, serving and interpretation of Decision Forest models in Keras. |